A user reported that they observed nodes in the cluster “going dark”. Basically, they would stop communicating with the rest of the cluster, but would otherwise appear functional. Both the internal and external metrics were all fine, the server would just stop responding to anything over the network. The solution for the problem was to restart the service (note, the service, not the whole machine), but the problem would happen every few days.
As you can imagine, we are taking this sort of thing very seriously, so we looked into the problem. And we came up short. The problem made absolutely no sense. The problem occurred on a (minor) version migration, but there was absolutely nothing related to this that could cause it. What was really weird was that the service itself continue to work. We could see log entries being written and it was able to execute scheduled backups, for example. It would just refuse to talk to us over the network.
That was super strange, since the network itself was fine. All the monitoring systems were green, after all. For that matter, the user was able to SSH into the system to restart the service. This didn’t match with any other issue we could think of. Since the user worked around the problem by restarting the server, we didn’t have a lead.
Then we noticed the exact same problem in one of our cloud instances, and there we have much better diagnostic capabilities. Once we had noticed a problematic server, we were able to SSH into that and try to figure out what was going on.
Here is what we found out:
- The server will not respond to HTTP(s) communication either from outside the machine or by trying to connect from inside the machine.
- The server will respond to SNMP queries both from inside the machine and outside of it (which is how we typically monitor the system).
When we designed RavenDB, we implemented a “maintenance hatch” for such scenarios, in addition to using HTTP(s) for communication, RavenDB also exposes a named pipe that allows you to connect to the server without going through the network at all. This ensures that if you have administrator privileges on the server, you are able to connect even if there are network issues, certificate problems, etc.
Here is the kicker. Under this particular situation, we could not activate this escape hatch. That is not supposed to be possible. Named pipes on Linux, where we run into the problem, are basically Unix Sockets. A network issue such as a firewall problem or something similar isn’t going to affect them.
At the same time, we were able to communicate with the process using SNMP. What is the problem?
Lacking any other options, we dumped the process, restarted the service, and tried to do the analysis offline. We couldn’t find any problem. All the details we looked at said that everything was fine, the server was properly listening to new connections and it should work. That was… weird.
And then it happened again, and we did the same analysis, and it came back the same. We were clueless. One of the things that we updated between versions was the .NET runtime that we were using, so we opened an issue to see if anyone ran into the same problem.
And then it happened again. This time, we knew that just looking at the dump wouldn’t help us, so we tried other avenues. Linux has a pretty rich set of knobs and dials that you can look at to see what was going on. We suspected that this may be an issue with running out of file descriptors, running out of memory, etc.
We tried looking into what is going on inside the process using strace, and everything was fine. The trace clearly showed that the server was processing requests and was able to send and receive data properly.
Wait, go through that statement again please!
It is fine? But the reason we are using strace is that there is a problem. It looks like the problem fixed itself. That was annoying, because we were hoping to use the trace to figure out what is going on. We added more monitoring along the way, which would let us know if the server found itself isolated. And we waited.
The next time we ran into the problem, the first thing we did was run strace, we needed to get the root cause as soon as possible, and we were afraid that it would fix itself before we had a chance to get to the root cause. The moment we used strace, the server got back online, continuing as if there was never any issue.
Over the next few instances of this issue, we were able to confirm the following observations:
- The service would stop responding to TCP and Unix Sockets entirely.
- There were no firewall or network issues.
- The service was up and functional, tailing the log showed activity.
- We could query the server state using SNMP.
- Running strace on the service process would fix the problem.
There are a few more things, the actual trigger for the fix wasn’t strace itself. It was the ptrace() call, which it uses. That would cause the service to start responding again. The ptrace() call is basically the beginning and the end of debugging under Linux. Everything uses it.
If you want to dump a memory process, you start with ptrace(). You want to trace the calls, ptrace(). You want to debug the process? GDB will start by calling ptrace(), etc.
And doing that would alleviate the problem.
That was… quite annoying.
We still had absolutely no indication of what the root cause even was.
We suspected it may be something inside Kestrel that was causing a problem. But that wouldn’t affect the named pipes / Unix sockets that we also saw.
Networking worked, because SNMP did. We thought that this may be because SNMP uses UDP instead of TCP, and looked into that, but we couldn’t figure out how that would be any different.
Looking at this further, we found that we have this in the code dumps:
~~~~ 5072
1 Interop+Sys.WaitForSocketEvents(IntPtr, SocketEvent*, Int32*)
1 System.Net.Sockets.SocketAsyncEngine.EventLoop()
1 System.Net.Sockets.SocketAsyncEngine+<>c.ctor>b__14_0(Object)
As you can see, we are waiting for this in the .NET Sockets thread. The SNMP, on the other hand, looked like:
Thread (0x559):
[Native Frames]
System.Net.Sockets!System.Net.Sockets.SocketPal.SysReceive()
System.Net.Sockets!System.Net.Sockets.SocketPal.TryCompleteReceiveFrom()
System.Net.Sockets!System.Net.Sockets.SocketAsyncContext.ReceiveFrom()
System.Net.Sockets!System.Net.Sockets.SocketPal.ReceiveFrom()
System.Net.Sockets!System.Net.Sockets.Socket.ReceiveFrom()
SharpSnmpLib.Engine!Lextm.SharpSnmpLib.Pipeline.ListenerBinding.AsyncReceive()
That was really interesting, since it meant that for sockets (both HTTP and Unix), we were always using async calls, but for SNMP, we were using the synchronous API. We initially suspected that this may be something related to the thread pool. Maybe we had something that blocked it, but it turns out to be a lot more interesting. Here is the code that is actually handling the SNMP:
var count = _socket.ReceiveFrom(buffer, ref remote);
Task.Factory.StartNew(() => HandleMessage(buffer, count, (IPEndPoint)remote));
In other words, we are actually reading from the socket in a blocking manner, but then processing the actual message using the thread pool. So being able to get results via SNMP meant the thread pool was well.
At this point we resulted to hair pulling, rubber ducking and in some instances, shaking our fists to heaven.
I reminded myself that I’m an adult with a bit of experience solving problems, and dug deeper. We started looking into how .NET is actually handling sockets in async mode. This end up here, doing a system call:
while ((numEvents = epoll_wait(port, events, *count, -1)) < 0 && errno == EINTR);
Reading through the man page for epoll_wait() I learned how epoll() works, that it is complex and that we need to be aware of level-triggered and edge-triggered options. Since .NET uses edge-triggered events (EPOLLET, which I keep reading as electronic chicken), we focused on that.
There are a lot of edge cases and things to cover, but everything we checked was handled properly. We finally had a good smoking gun. For some reason, we weren’t getting notifications from epoll(), even though we should. Using strace() or friends somehow fixes that.
We actually found the exact scenario we saw in StackOverflow, but without any idea what the issue was. Truly, there is an XKCD for everything.
Our current understanding of the issue:
- All async sockets in .NET are going through the same socket engine, and are using epoll() under the covers.
- SNMP is using synchronous calls, so it wasn’t using epoll().
That covers both of the weird things that we are seeing. So what is the issue?
It is not in .NET. Given the size & scope of .NET, we wouldn’t be the only ones seeing that. Below .NET, there is the kernel, so we looked into that. The machines we were running that on were using kernel 5.4.0-azure-1095, so we looked into that.
And it looked like it is a kernel bug, which was fixed in the next updated kernel. A race condition inside the kernel would cause us to miss wakeups, and then we would basically just stall without anything to wake us up.
We dug deeper to understand a bit more about this situation, and we got this:
Some system calls return with EINTR if a signal was sent to a
tracee, but delivery was suppressed by the tracer. (This is very
typical operation: it is usually done by debuggers on every
attach, in order to not introduce a bogus SIGSTOP). As of Linux
3.2.9, the following system calls are affected (this list is
likely incomplete): epoll_wait(2), and read(2) from an inotify(7)
file descriptor. The usual symptom of this bug is that when you
attach to a quiescent process with the command
strace -p <process-ID>
then, instead of the usual and expected one-line output such as
restart_syscall(<... resuming interrupted call ...>_
or
select(6, [5], NULL, [5], NULL_
('_' denotes the cursor position), you observe more than one
line. For example:
clock_gettime(CLOCK_MONOTONIC, {15370, 690928118}) = 0
epoll_wait(4,_
What is not visible here is that the process was blocked in
epoll_wait(2) before strace(1) has attached to it. Attaching
caused epoll_wait(2) to return to user space with the error
EINTR. In this particular case, the program reacted to EINTR by
checking the current time, and then executing epoll_wait(2)
again. (Programs which do not expect such "stray" EINTR errors
may behave in an unintended way upon an strace(1) attach.)
And.. that is exactly what is happening. On attaching, the epoll_wait() will return with EINTR, which will cause .NET to retry the command, and that “fixes” the issue.
It makes total sense now, and concludes the discovery process of a pretty nasty bug.
Now, if you’ll excuse me, I need to go and apologize to a rubber duck.
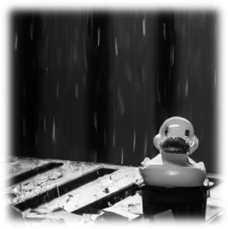