The Sled project is an embedded database written in Rust. I run into it a few times recently and given my day job, I decided to take a peek and understand how it works. The project talks about being Log Structure Merge (and also exposing this to the client) with B+Tree read performance. The last time I read an LSM codebase was quite some time ago, so this is going to be quite interesting, I hope.
As usual, I’m doing a stream of consciousness as I’m going through the code. I’m looking at commit 6ce3e1264d3bb46de768e8fe338bade02d0b30dc.
The first thing that I found is the actual source code for this:
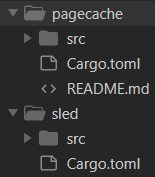
The fact that there is a page cache component already tell me quite a lot about the project. That is uses pages (LevelDB does not, for example) and that it is cached (so likely not using mmap). That will likely be important later, but when starting out I enjoy jumping to conclusions and then seeing if I was right or not.
The following is taken from the readme for the pagecache:
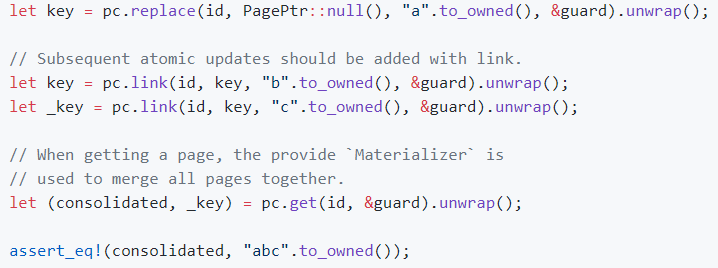
This is quite interesting. Mostly because this shows a very different approach to building a page cache. It isn’t your usual B-Tree page, it seems. The docs reference LLAMA a lot, but I would rather go through the code first and read scholarly articles later.
As usual, I’m going over the code in lexical order, which means that the first real code file that I run into is a Rust implementation of doubly linked list. Nothing really interesting there, except massive amounts of unsafe code, so I’m going to skip to it’s usage, which is to implement a least recently used cache.
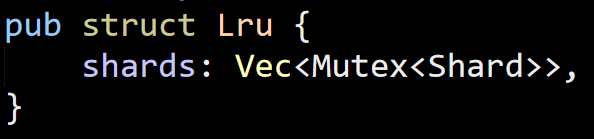
There are N shards, determined by the configuration of the system. This is controlled by cache_bits, where 2^CacheBits is the actual size. I’m not sure why the code uses this methods instead of just a number.
The codebase contains the values 0, 2 and 6 for cache_bits, giving us 1 shard, 4 shards and 64 shards, respectively. I assume that they use this to reduce contention on the same Shard instance for concurrent operations.
The LRU’s main method is accessed, which simply forward the call to one of the shards, so I’m going to skip that and see how that actually works when I’ll look at the shard code. Here is what the Shard looks like:
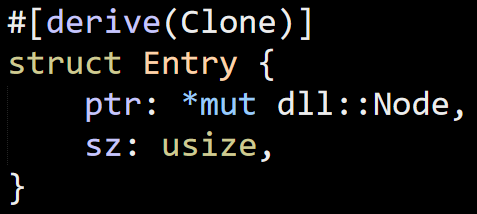
Note that capacity is actually the max capacity, not reserved space in the entries vector. Each entry contains the actual size that it is using, as well and the Node itself (that reside in the LRU). The actual value that is kept is a PageId, which is the native integer type for the platform (32 bits – 4 bytes / 64 bits – 8 bytes). I’m not sure yet if this is a persisted value, but for something that is meant to be used in a persisted store, I find that choice very strange. It means that on 32 bits machine, you can only use 4 billion pages, while 64 bits there is much higher limit. That means that a database created on 64 bits might not be valid for 32 bits.
When building Voron, we were very careful that data will be the same in 32 bits and 64 bits, Windows / MacOS / Linux, etc.
When you call accessed on the Shard, it will add it to the list, and if the size of all entries is higher than the capacity, will remove entries until the size in the cache is smaller than the maximum capacity.
Interestingly enough, Sled is using an Epoch based GC system in Rust, implemented by Crossbeam. I wrote about Epoch systems when I reviewed FASTER, and I understand that Epoch GC is pretty common in Rust concurrent programming, because otherwise you run the risk of memory corruption.
The ds (I assume data structure) folder of the page cache also provide a concurrent Stack implementation, which it pretty elegant to read, but you need to be well versed in the Epoch handling to understand how it all works correctly under the covers.
The interesting stuff in the ds folder is the pagetable, which start with the following structs:
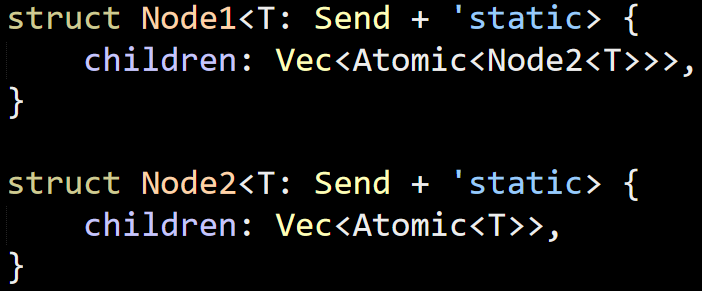
Each of those is initialized to array of pointers that is 2 MB in size (262,144 pointers, basically). That is a pretty big structure, and the PageTable struct ties it all together:
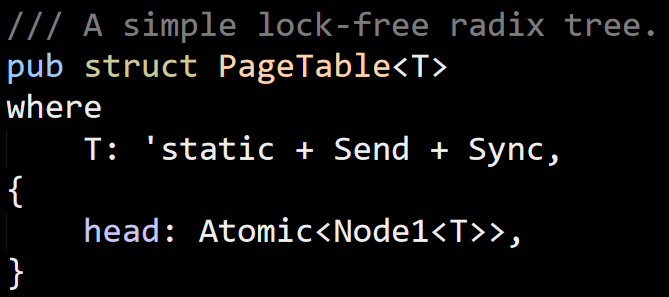
The top of the file mention that this assume that the caller is going to have a dense key space, and this make sense. Theoretically, this will use a maximum of 512GB if you store a sparse set of data in the page table.
All the operations in the page table are some variant of the traverse operation, so let’s focus on that.
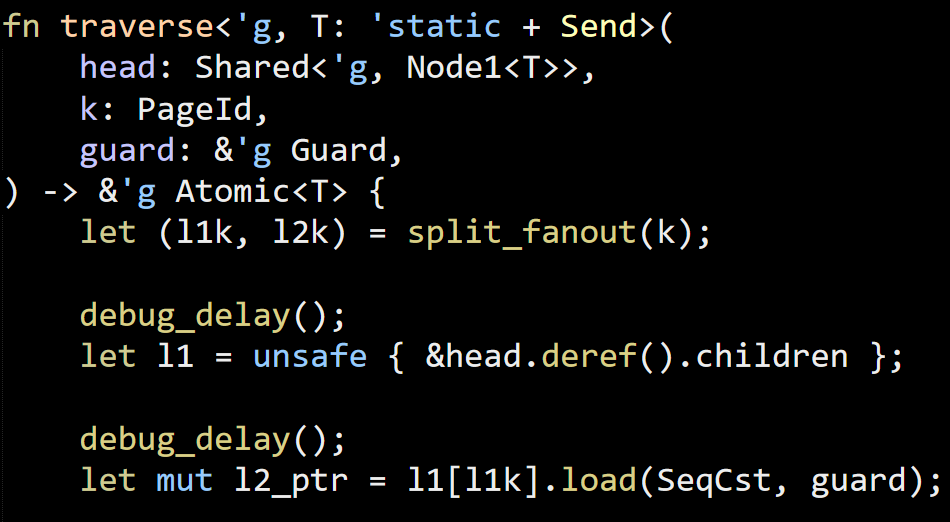
First, the key is split to the first 18 bits (0 .. 256K) and the rest of the value. The first part is used to find the level 1 value.
The debug_delay() calls are debug only calls that add random waits, which help catch race conditions. Given that the code uses explicit Atomic instructions, I’m not surprised to see that there is really no help from the Rust safety behavior to ensure that there aren’t any logical race conditions.
This is the first time I’m reading real Rust code that explicitly deal with concurrency (without using the hammer that is Mutex, at least), and it is… reassuring seeing the same kind of behaviors that I’m injecting into my code to validate things.
This next bit handles adding a new value (safely) at the next level. Given the dense assumption, we can safely assume that this is a rare operation
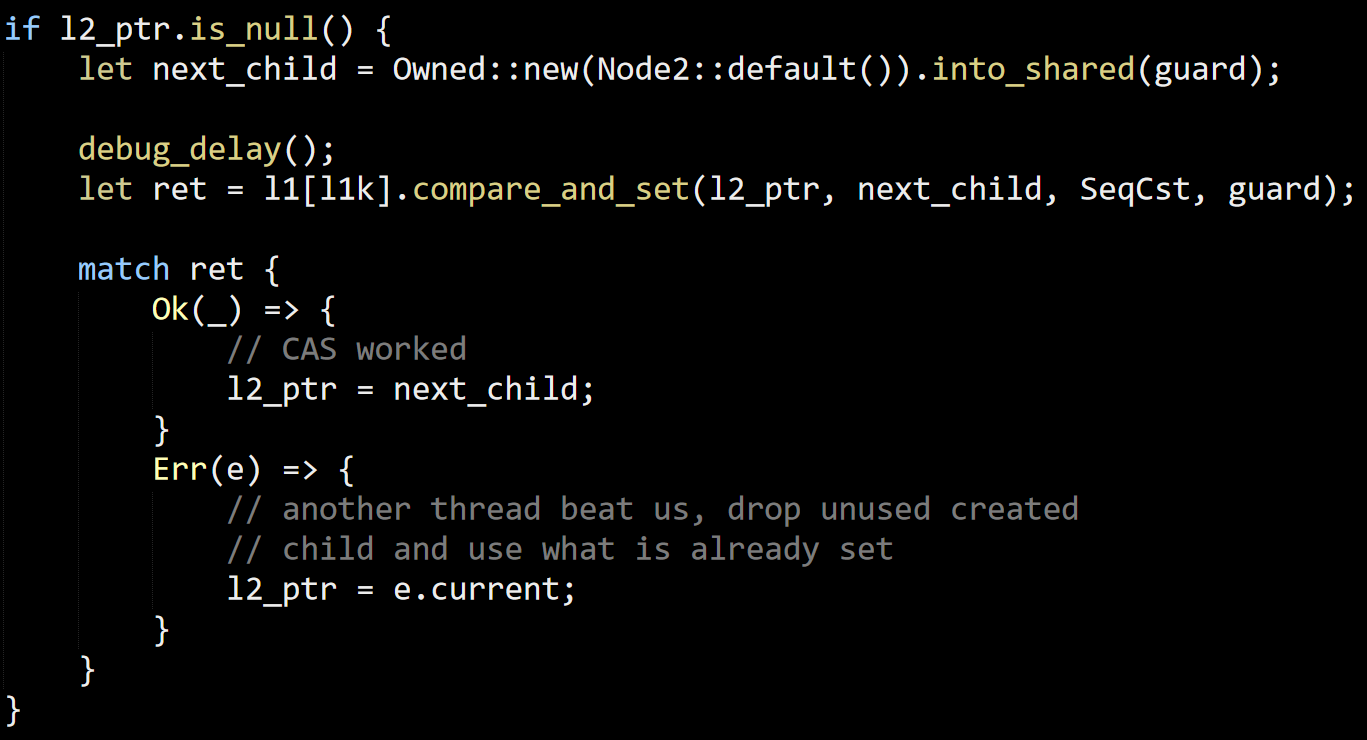
Look at the comment when we fail to win the compare_and_set call. Who is actually going to drop the unused created value? That is on the Guard and Epoch, I think. We associated the value with them when we called into_shared, and since we didn’t take it out from the guard, it will be removed when the Epoch is over.
The final act of traverse() is to just return the value from the second level, like so:
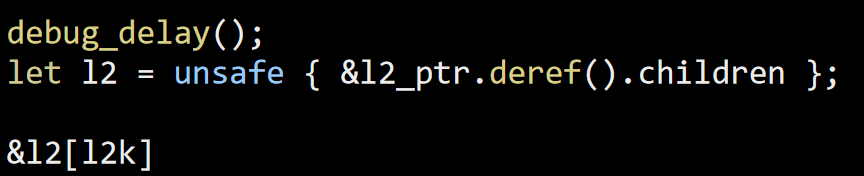
Note that this may be an empty value, so let’s see how this actually get used. I’m using the simplest caller, which is get().
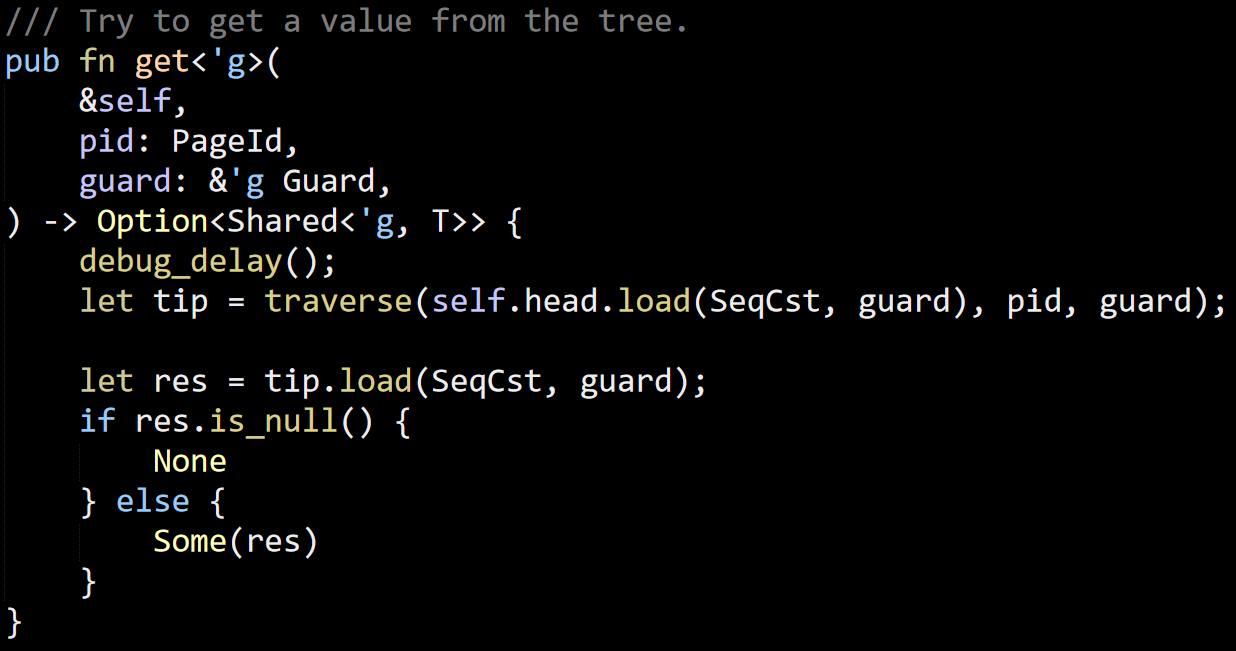
You can see that we have to do explicit null checking, translating that to an Option call to make calling code idiomatic. The rest of the code there is just memory management, so I’m going to skip that and look at the rest of the pagecache code, now that I understand the underlying data structures.
Note, the code uses the Lsn as a logical type name, this maps to a 64 bits signed integer.
The first file in the pagecache crate is the blob_io one, which handles reading blob. Here is the (crate public only) functions that are implemented there.

Remember, LSN is the (Logical Sequence Number) and is a signed 64 bits integer. Config I’m not sure about at this point. The read_blob and write_blob are fairly simple. They just read / write (with CRC32 checks) data from disk. This is done on a full file each time. So that is quite interesting. The Config is responsible for mapping between an LSN and a file path.
The call to remove_blob will simply delete the mapped file from the disk, but it is the call to gc_blobs that is really interesting. What this does is to basically delete all the files whose name is greater than the stable_lsn provided. This is interesting, because it tells us a lot about the actual on disk persistent format.
Based on just the blob_io.rs file, I can tell that Sled is writing fairly large files to disk and reading them to memory. It is probably going to call gc_blobs() as part of its recovery and I’m willing to assume at this point that each blob is actually a segment. As I said, I like assuming things upfront when doing this kind of code reviews. I’m going to continue my reading and see if I was right.
The config file starts with usage instructions, which looks like:
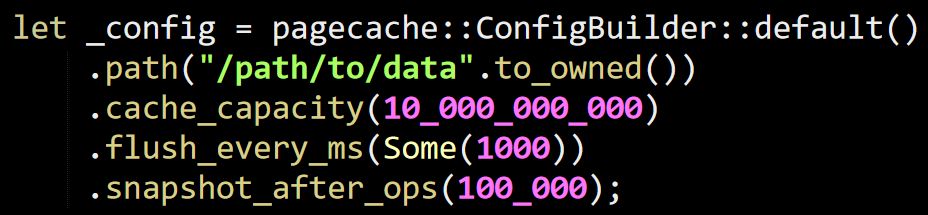
So that is really interesting. First, we can see that Sled is probably only calling fsync once in a while, depending on its configuration. I looked into a real world project, and it uses the default value, which is flush every 500 ms and snapshot after 1 million. The reason this is interesting is that my experience tells me is that whenever I see a configuration option like that, it means that fsync is going to be pretty expensive and the database engine has chosen to allow users to trade performance for safety.
Voron, in contrast, actually don’t have any such feature. We don’t allow users to make that choice, instead, we accepted the fsync costs and dealt with them (using async commit and transaction merging, for example).
Anyway, we were talking about the config and how it works, here is how the Config gives us the path for a blob:
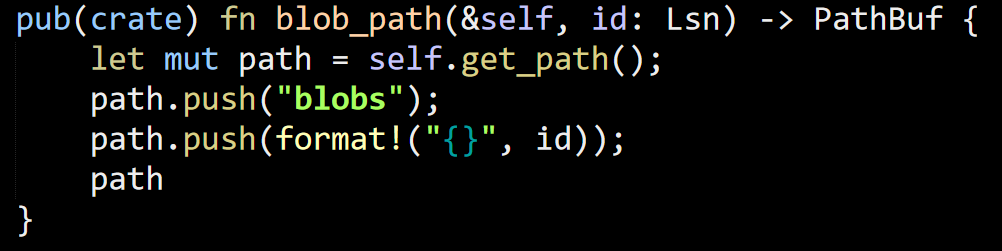
So this is pretty much just as expected. The data for each blob is a separate file under the blobs directory. Now we are only left with figuring out what that blob was.
The next file of interest is the DiskPtr file, which contains this struct:
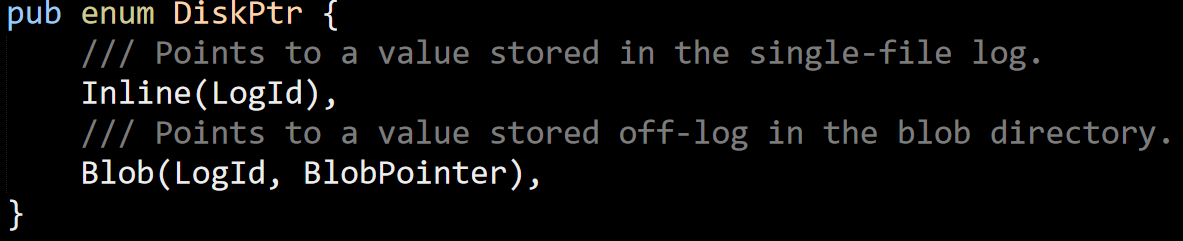
And the only interesting method is the read():
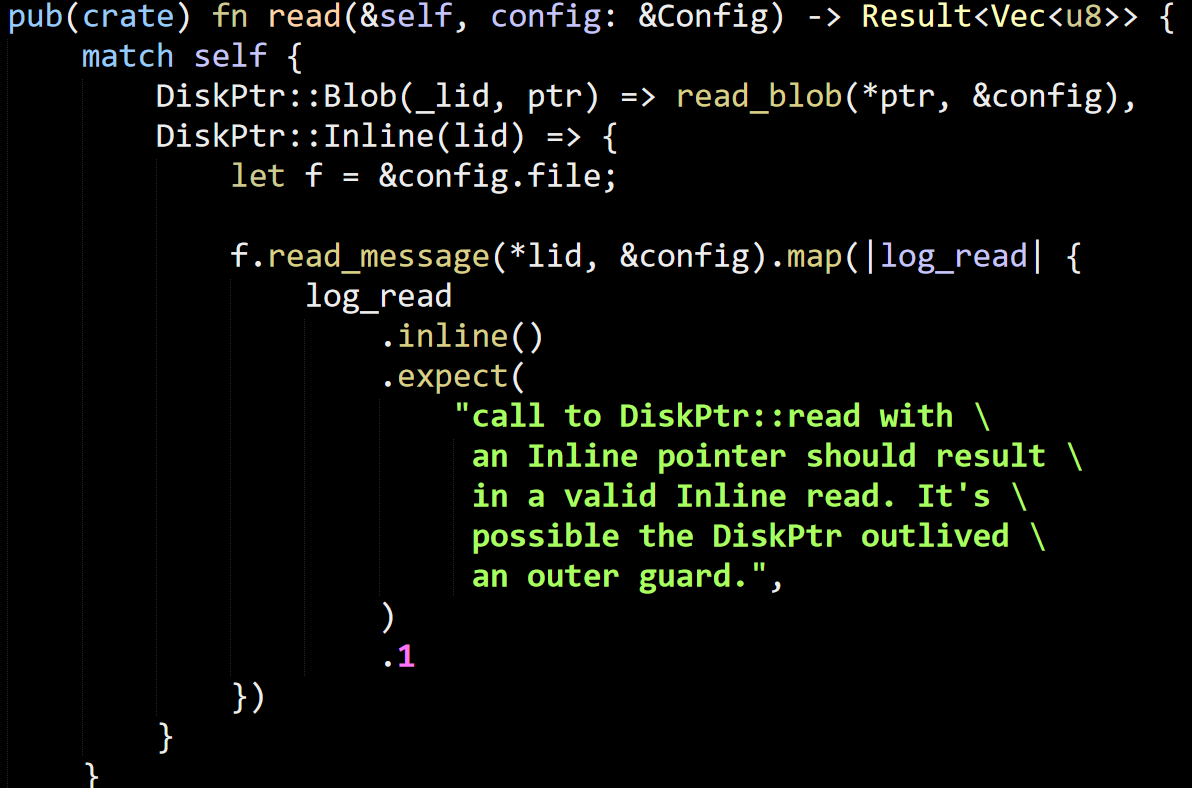
So now we have more interesting data. There is stuff that is kept inline (presumably small items) and larger stuff that are read from the blobs directory. We already saw what the read_blob behavior is, but we haven’t checked what the meaning of read_message is. This is implemented later in the code, so I’m going to ignore it and just look at its return value:
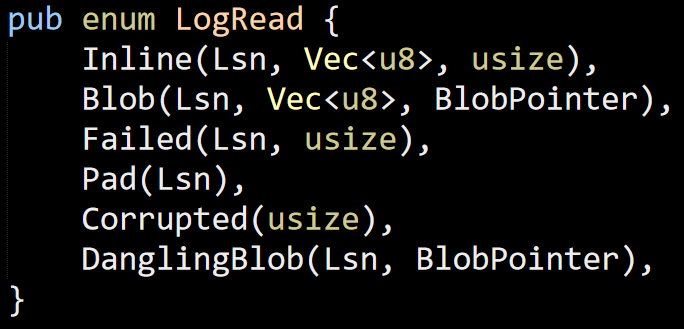
So that allow us to make sense of what is going above. Basically, this is “gimme some bytes” call, regardless of where this is actually stored (which I don’t know yet).
The next file is event_log, and it is a debug tool to help verify the behavior of the system. I wholeheartedly approve and it is a really good sign for the maturity of the codebase.
The next is flusher, which is a dedicate thread that call this continuously:

There is nothing of interest in there, but it does indicate no coordination between flushing and other activities. Not sure whatever this is a good or bad idea.
Now, let’s see what this iobuf is all about, shall we?
The first thing I run into in this file is this:
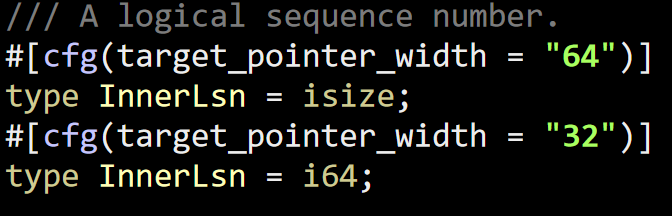
And I can’t really figure this one out. I mean, why? Just setting InnerLsn = i64 should be good enough for all platforms, I think. I guess there might be some optimizations stuff that I’m missing, but that seems unlikely. In 64 bits, I would expect isize and i64 to have the exact same behavior.
This is now getting pretty late, so I’m going to close this for today. Tomorrow, I’m going to try to figure out how the rest of it works.