Okay, so far I have written 6 parts, and the only thing that happened is that we wrote some stuff to the log file. That is cool, but I am assuming that there has got to be more. I started tracking the code, and I think that what happens is that we have compactions of the MemTable, at which point we flush it to disk.
I think that what happens is this, we have a method called MaybeScheduleCompaction, in db_impl.cc, which is kicking of the actual process for the MemTable compaction. This is getting called from a few places, but most importantly, it is called during the Write() call. Reading the code, it seems that before we can go to the actual compaction work, we need to look at something called a VersionSet. This looks like it holds all of the versions of the database at a particular point in time. Including all the files that it is using, etc.
A lot of what it (and its associate, the Version class) is about managing lists of this structure:
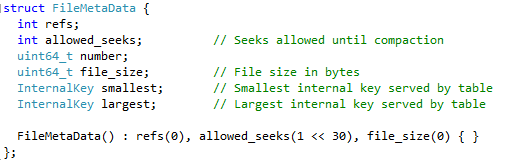
I am not sure what allowed_seeks mean, I assume it is there to force compaction for the next level.
Okay, moving on to version, it looks this is where all the actual lookups are done. We have a list of file metadata, including smallest & largest keys in each file. That allows us to find the appropriate files to look at quite easily. There seems to be some interaction between Version and TableCache, but I’m not going into that now.
A version is holding an array of 7 levels, and at each level we have the associated files. I am going to continue digging into Version & VersionSet for the moment.
Side Note: In fact, I got frustrated enough with trying to figure out leveldb on Windows that I setup a Ubunto machine with KDevelop just to get things done. This blog post is actually written on the Ubunto machine (later to be copy into live writer :-)).
I am still in the process of going through the code. It is a really much easier to do this in an IDE that can actually build & understand the code.
Once thing that I can tell you right now is that C++ programmers are strange. I mean, take a look at the following code, from Version::LevelFileNumIterator :
This returns a byte array containing encoded file num & size in a buffer. Would it be so hard to create a struct for that or use std::pair ? Seems like this would complicate the client code. Then again, maybe there is a perf reason that I am not seeing?
Then again, here is the client code:
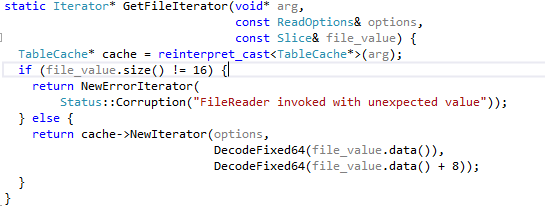
And that seems pretty clear.
So far, it appears as if the Version is the current state of all of the files in a particular point in time. I think that this is how leveldb implements snapshots. The files are SSTables, which are pretty much write once only. A version belong to a set (not sure exactly what that means yet) and is part of a linked list. Again, I am not sure what is the purpose of that yet.
I'll need to do a deeper dive into snapshots in general, later on, because it is interesting to see how that is implemented with regards to the memtable.
Moving back to the actual code, we have this code:
This seems to me to indicate that the table_cache is the part of the code that is actually manages the SSTables, probably using some variant of the page pool.
Now, let us get to the good parts, Version::Get:
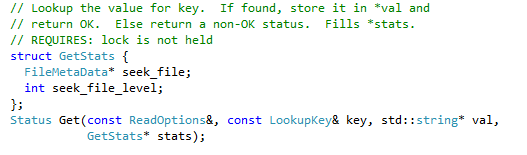
This looks like this is actually doing something useful. In fact, it find the relevant files to look for that particular key, once it did that, it calls:
So the data is actually retrieved from the cache, as expected. But there was an interesting comment there about “charging” seeks for files, so I am going to be looking at who is calling Version::Get right now, then come back to the cache in another post.
What is interesting is that we have this guy:
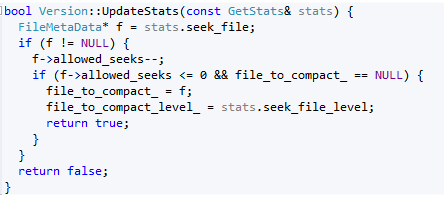
And that in turn all make sense now. allowed_seeks is something that is set when we apply a VersionEdit, it seems. No idea what this is now, but there is a comment there that explains that we use this as a way to trigger compaction when it is cheaper to do do compaction than continue doing those seeks. Interestingly enough, seeks are only counted if we have to go through more than one file to find a value, which makes sense, I guess.
Okay, now let us back up a bit and see who is calling Version::Get. And as it turned out, it is our dear friend, DBImpl::Get().
There, we first look in the current memtable, then in the immutable memtable (which is probably on its way to become a SSTable now. And then we are looking at the current Version, calling Version::Get. If we actually hit the version, we also call Version::UpdateStats, and if we need to, we then call MaybeScheduleCompaction(), which is where we started this post.
And... that is it for this post, we still have managed to find where we actually save to disk (they hid it really deep), but I think I'll probably be able to figure this out in this sitting, watch out for the next post.